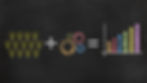
AIClub students had an amazing showing at the 2022 JSHS (Junior Science and Humanities Symposium). Three AIClub students entered at the regional level, in the Northern California, Southern California, and Virtual Regions. The students submitted novel research in Astronomy (classifying planetary objects), Climate (detecting greenhouse gas emissions), and Healthcare (forecasting health deterioration for brain cancer patients). All three students qualified for the National Symposium for which only 2% of all the regional submissions qualified! Two students - Aryan and Meenakshi - won their respective categories at the National level for their Climate and Astronomy Research!
What is the Junior Science and Humanities Symposium?
The Junior Science and Humanities Symposium (JSHS) is an annual US research competition for high school students. Students present research in a range of STEM fields to a panel of judges and can win recognition and scholarships. JSHS is a great way for aspiring high schoolers to learn about and practice research, and get feedback from professionals from university professors to government researchers.
How prestigious is the JSHS?
The JSHS is one of the most prestigious STEM competitions in the United States.
How many people compete in JSHS?
Each year over 12,000 high school students around the country participate in regional JSHS events at forty-eight regional symposia held on university campuses nationwide. This year, 245 of these students (about 2%) were selected to compete in the national event.
Does JSHS give you an advantage in college admissions?
Demonstrating innovation and being recognized for research, in both research competitions and science fairs, is a great way to stand out in college admissions at selective colleges.
Our JSHS 2022 National Winners
Aryan developed a novel technique for detecting and assessing greenhouse gas emissions from satellite imagery. His project abstract (taken from the JSHS website) is below:
Greenhouse gasses (GHG) emitted from fossil-fuel-burning power plants pose a global threat to public health, causing 8.7 million deaths per year through air pollution and raising the frequency of natural disasters. Quantifying GHG emissions is crucial for the success of future climate action. However, current methods to track emissions cost upwards of $520,000 per power plant. These methods are cost prohibitive for developing countries, and are not globally standardized, leading to inaccurate estimations in emissions reports from nations and companies. I developed a novel, low-cost solution via a end-to-end deep learning pipeline that utilizes observations of emitted smoke plumes in satellite imagery to provide an accurate, precise system for quantifying GHG emissions at an global scale by 1) segmentation of power plant smoke plumes 2) classification of the type of fired fuel 3) algorithmic prediction of power plant CO2 emissions. The pipeline was able to achieve a segmentation Intersection Over Union (IoU) score of 0.924, fuel classification accuracy of 96%, and quantify power generation and CO2 emission rates with a R-Squared (R2) value of .91 and a Mass Absolute Error (MAE) within 6.3%, indicating high performance across global regions. The results of this work are significant because they enable the identification of major sources of GHG emissions and their temporal monitoring on a global scale at a low-cost. This enables the development of more-effective climate policy and transparency regarding compliance with the Paris Climate Agreement and COP26 goals, revolutionizing the way we tackle climate change.
Meenakshi developed a novel hybrid machine learning/deep learning method to classify planetary nebulae from imagery from the Hubble Space telescope. Her project abstract (taken from the JSHS website) follows:
There are believed to be ~20,000 nebulae in the Milky Way Galaxy; however, humans have only cataloged ~1,800 of them even though we have gathered 1.3 million observations. Resources like the Hubble telescope can automatically explore space and discover new artifacts. Still, their classification is a human skill, which ultimately is interminable and subject to human error. The importance of classifying nebulas cannot be stressed enough. Studying the chemical composition of a nebula can help us understand the material of the original star. My research of nebulae classification aims to make the process of discovering and classifying new nebulae faster and more accurate using a hybrid of Deep Learning and Machine Learning techniques. Nebulae can be classified into five different categories: planetary, supernova remnants, emission, reflection, and dark. Using a dataset primarily of images from the European Space Agency, I experimented with a range of artificial intelligence techniques and determined the deep learning network/machine learning algorithms that produced the best results. Some experiments included converting the images to black and white, featurization, and dropping specific categories. The main conclusions reached from my research were that the AI was not dependent on color to classify nebulae, dropping specific categories increased accuracy and that featurization is the most effective technique to classify nebulae accurately. The discovery of new astronomical bodies is already mechanized. Making the classification of nebulae automated will help us discover, identify, and classify these marvels much faster and accurately.
Congratulations to all!
If you would like to learn more about how to do research projects like the ones above, please contact us at info@pyxeda.ai for a consultation.